At the Warren B. Nelms Institute for the Connected World, located in the Herbert Wertheim College of Engineering on the University of Florida campus, My T. Thai, Ph.D., a professor in the Department of Computer & Information Science & Engineering and associate director of the Institute, is developing software technologies that can explain how bias can creep into artificial intelligence (AI) algorithms. Her research is helping users who work with AI technology to extract analyses and predictions that are accurate and as close to reality as possible, as opposed to nominal, or worse, prejudiced and unfair.
Bias creep occurs when data and its patterns are misread and analyses become tainted. Increasingly, industries and policy-makers rely on AI to tackle voluminous and rapid data processing. If the AI learns and informs from a pattern that is incorrectly interpreted, the result could lead to serious errors in decision-making and response-formulation.
Language bias can be an especially significant factor in incorrect data interpretation, especially in venues such as career fairs for college students. For example, at a recent UF Department of Electrical & Computer Engineering career fair, a recruiter advised students to look at the job description carefully and ensure that their CV had certain keywords in it. “It would be a shame if the AI rejects you before you even get the chance to talk to someone,” the recruiter said. The language bias in the machine learning program may have trained the AI to take a shortcut and reject a CV that does not contain specific words, even though the student might be eminently qualified for the job.
Dr. Thai, funded by a grant from the National Science Foundation (NSF) and working with industry partner Amazon, is currently developing software technologies to help identify and explain where and how bias enters AI algorithms. “Using AI models as ‘black boxes’, without knowing why the model made a particular decision about the data, degrades the trustworthiness of the system,” Dr. Thai said. If the AI begins to use shortcuts, such as discounting any data that is not provided in a specific format designated by the machine learning tools used to train it, bias can begin to creep into the AI technology.
“We can use the models we are developing to explain ‘shortcuts learning’ in the machine learning tools used to train the AI,” Dr. Thai said. “Not only will this type of explainable machine learning help AI users improve the fairness of their systems and technologies, it will provide them with a tool for demonstrating transparency in their operations. Without the ability to explain bias in the data or the machine learning tools, there is no transparency, which will limit the usefulness of the results.”
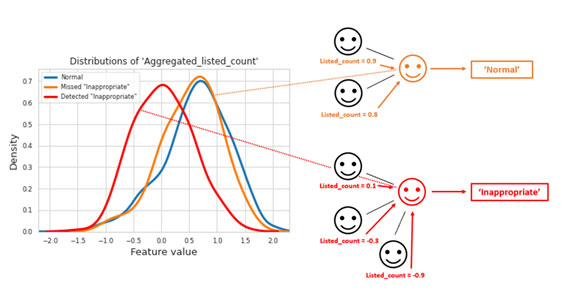
Shortcuts learning was initially identified using images and text data, but now Dr. Thai and her students have exposed a new type of shortcuts learning in Graph Neural Networks (GNNs), a novel deep learning technique based on graph data. Using her recently developed explainable machine learning model, Probabilistic Graphical Model Explanations (PGM-Explainer), on a GNN that had been trained to classify ‘inappropriate’ users who endorse hate speech on Twitter, Dr. Thai and her group learned that the AI model relied on the shortcut “aggregated-listed-count” to generate a prediction. A high value for aggregated-listed-count indicated that the user had many important neighbors belonging to a high number of Twitter lists. Yet, this characteristic does not hold a causal effect on deciding whether the user is ‘inappropriate’ or not. It is merely a shortcut exploited by the AI model to correlate and erroneously classify some ‘inappropriate’ users as ‘normal’. The PGM-Explainer shows the shortcut was learned by the model and, therefore, the results are not reliable.
When Dr. Thai completes her current work, the explainable machine learning code will be available for licensing by companies and organizations. Working with the code to assess AI biases will require employees with basic training in AI. This need complements the AI workforce development vision highlighted in the UF AI University initiative. In making artificial intelligence the centerpiece of a major, long-term initiative that combines world-class research infrastructure, cutting-edge research, and a transformational approach to curriculum, the goal is to equip every UF student with a basic competency in AI regardless of their field of study. Furthering lifelong learning opportunities for every citizen of Florida is also a goal under this initiative, so that Florida will become the AI technology talent pipeline for the State and the country.
“The work Dr. Thai is doing will help advance the effectiveness of AI and IoT in all fields of academe, commerce and industry,” said Swarup Bhunia, Ph.D., Semmoto Endowed Professor and Director of the Warren B. Nelms Institute for the Connected World. “This is exactly what the Nelms Institute was established for: to lead research and education in all aspects of the intelligent connection of things, processes and data that address major world challenges. And right now, the world deserves a better AI technology that ensures fairness.”
Diane Choate
Public Relations & Communications Strategist
Herbert Wertheim College of Engineering