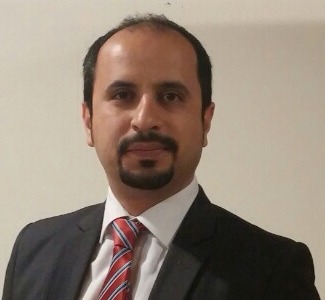
Date: October 11, 2019
Time: 11:00 AM - 12:00 PM
Location:
444 Newell Drive, Gainesville, FL, 32611
Host: UF CISE Department
Admission: This event is free and open to the public.
Protein Fold, Local Structure, and Post Translational Modification Predictions Using Machine Learning
Abstract: Proteins are the most important biological macromolecules which play a wide range of vital roles in biological interactions. The function of proteins relies on how they fold in 3D space which is referred to as their tertiary structure. Predicting tertiary structure of a protein from its amino acid sequence (also called the primary structure) is considered to be one of the most challenging unsolved problems in science. Hence, there is a critical need to propose a fast, cost effective computational toolkit to address this problem. The objective of my research is to build an integrated, multi-purpose, and accurate integrated machine learning-based model to predicts the most important protein structure prediction sub-problems including protein fold, structural class, subcellular localization, and post translational modification (PTM). To do this I employ different classification techniques with the focus on deep learning architecture trained on the novel features extracted and selected based on evolutionary and structural properties of proteins. I am going to discuss my previous and current research in building such integrated model and my future direction to achieve this goal.
Biography: Iman Dehzangi, Ph.D., is an assistant professor at Morgan State University’s Department of Computer Science. He is also the director of MS in Bioinformatics program at MSU. Before coming to MSU, he was a Postdoctoral research scholar at the University of Iowa. He received his PhD in computer science (majoring in Machine Learning, Bioinformatics and Computational Biology) from Griffith University at the Institute for Integrated and Intelligent Systems. His research focus is on machine learning, artificial intelligence, and bioinformatics & computational biology in general. He works on the challenges associated with the design and development of robust, general, and accurate systems for several important problems in these fields such as, protein local and global structure prediction, genome variants analysis, and cancer subtype classification, in specific. His main focus is on using machine learning techniques with emphasis on deep learning architecture, support vector machines, and ensemble classifiers to tackle these problems.